The AFL-CIO/Cornell-ILR Union Leadership Institute prepares labor professionals to advance workers’ rights in New York state. |
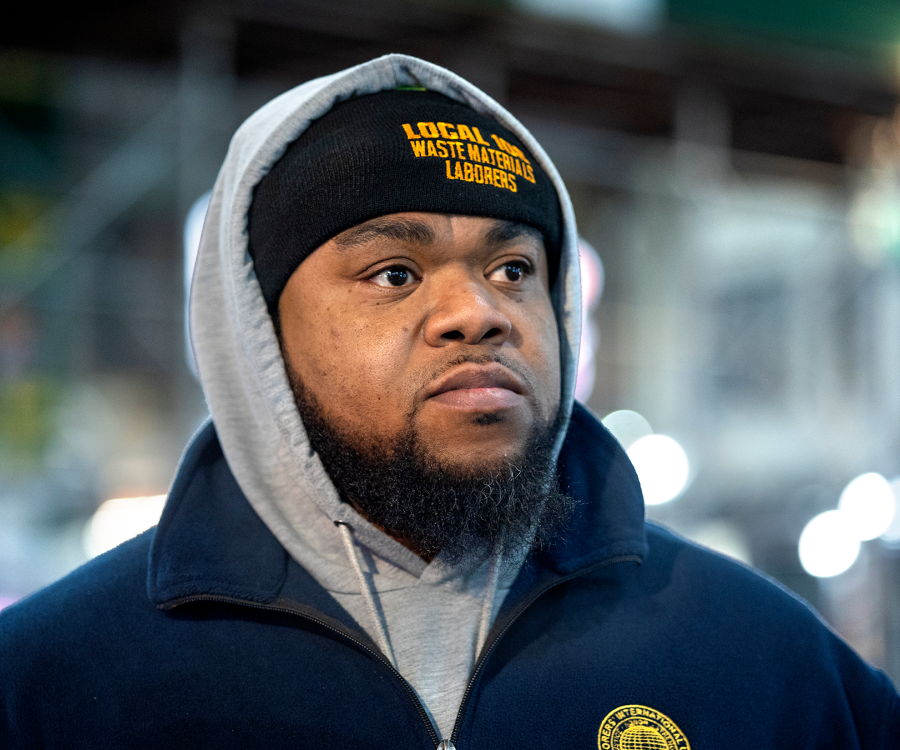
ILR School Events
See all eventsTrevon Logan, Ohio State Competition and Consumer Discrimination in Public Accommodations Abstract: In models of consumer discrimination, discrimination can persist in equilibrium. We present a model of discrimination where one group of consumers have discriminatory preferences related to consuming alongside another group of consumers. The model identifies the equilibrium relationship between the ratio of consumers of both types and the ratio of non-discriminatory to discriminatory firms in a local market. We examine this empirically using a new county-level dataset constructed from the Negro Motorist Green Books and the Census of Business to measure the number of non-discriminatory and discriminatory public accommodations in the United States between 1939 to 1955. Using various sources of plausibly exogenous variation in the consumer population ratio, we show that changes in the racial composition of consumers led to increases in the ratio of discriminatory to non-discriminatory firms in the post-war era. We also show a strong role for market power, where increasing provision of non-discriminatory treatment was primarily seen in the least competitive markets. Using novel data on prices matched to firms, we also show that since far more firms were in the discriminatory market than the non-discriminatory market, the prices in the discriminatory market were not higher than in the non-discriminatory market. The results imply that consumer preferences for discrimination were remarkably strong historically, that market power blunted the influence of consumer preferences, and that extensive racial discrimination would have been maintained nationwide without bans on racial discrimination in public accommodations.
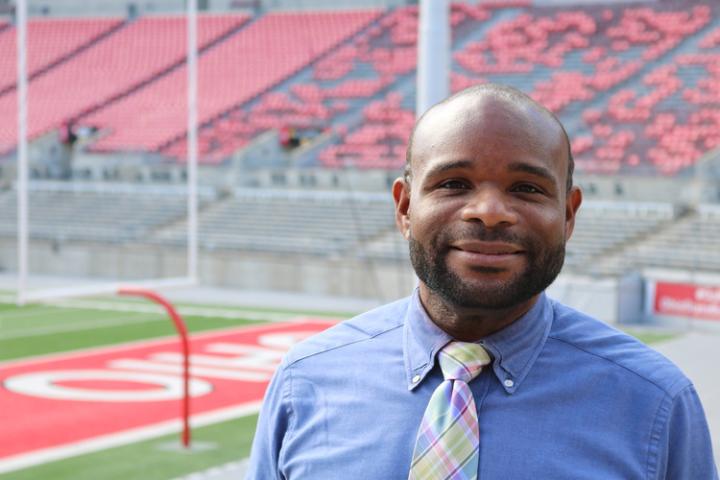
Edoardo Teso, Northwestern State Capacity as an Organizational Problem. Evidence from the Growth of the U.S. State Over 100 Years Abstract: We study how the organization of the state evolves over the process of development of a nation, using a new dataset on the internal organization of the U.S. federal bureaucracy over 1817-1905. First, we show a series of novel facts, describing how the size of the state, its presence across the territory, and its key organizational features evolved over the nineteenth century. Second, exploiting the staggered expansion of the railroad and telegraph networks across space, we show that the ability of politicians to monitor state agents throughout the territory is an important driver of these facts: locations with lower transportation and communication costs with Washington DC have more state presence, are delegated more decision power, and have lower employee turnover. The results suggest that high monitoring costs are associated with small, personalistic state organizations based on networks of trust; technological innovations lowering monitoring costs facilitate the emergence of modern bureaucratic states.
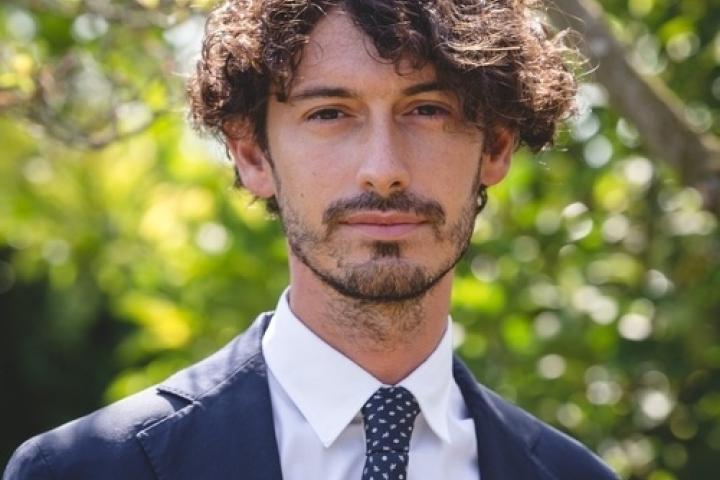
Future of Work Fellowships
The ILR Future of Work fellowship program supports postdoctoral researchers and doctoral students who work with our world-leading faculty on innovative and impactful research projects.
This fellowship program is designed to promote the benefits of strong collaboration between newer researchers and resident faculty members in studying impactful topics related to the future of work. Fellows and their faculty sponsors alike are enabled to address challenging research questions and break out of any stereotypes or default thinking around the future of work.
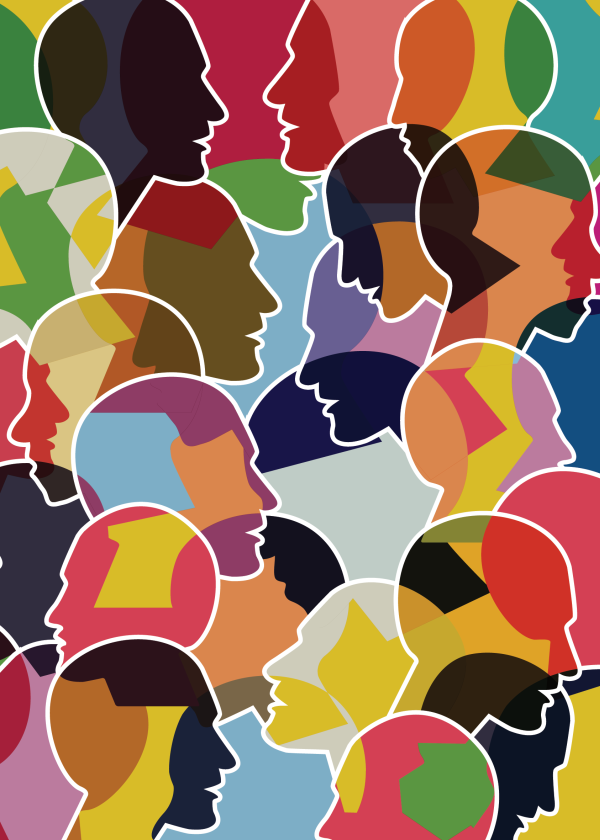
Professor Martin Wells has been elected a fellow of the American Association for the Advancement of Science (AAAS), the world's largest general scientific society.
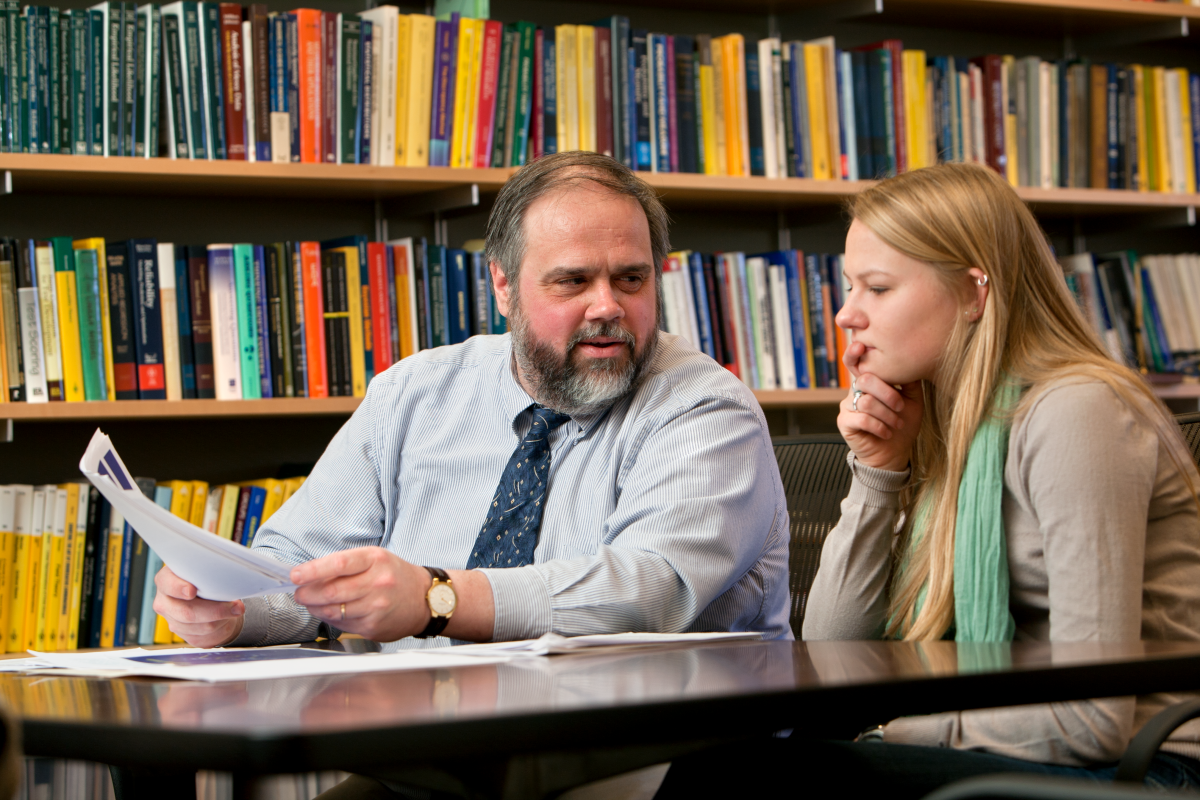
ILRies Change
the Future of Work.
The Martin P. Catherwood Library is the most comprehensive resource on labor and employment in North America, offering expert research support through reference services, instruction, online guides and access to premier collections.
